报告时间:10月9日(星期五)下午15:00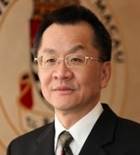
报告地点:南望山校区八角楼学术报告厅
报 告 人:陈俊龙教授 华南理工大学(South China University of Technology)
报告题目:A Novel Efficient RNN and LSTM-Like Architecture: Recurrent and Gated Broad
Learning Systems and Their Applications
报告人简介:C. L. Philip Chen is the Chair Professor and Dean of the College of Computer Science and Engineering, South China University of Technology. Being a Program Evaluator of the Accreditation Board of Engineering and Technology Education (ABET) in the U.S., for computer engineering, electrical engineering, and software engineering programs, he successfully architects the University of Macau’s Engineering and Computer Science programs receiving accreditations from Washington/Seoul Accord through Hong Kong Institute of Engineers (HKIE), of which is considered as his utmost contribution in engineering/computer science education for Macau as the former Dean of the Faculty of Science and Technology. He is a Fellow of IEEE, AAAS, IAPR, CAA, and HKIE; a member of Academia Europaea (AE), European Academy of Sciences and Arts (EASA), and International Academy of Systems and Cybernetics Science (IASCYS). He received IEEE Norbert Wiener Award in 2018 for his contribution in systems and cybernetics, and machine learnings. He is also a highly cited researcher by Clarivate Analytics in 2018 and 2019.
His current research interests include cybernetics, systems, and computational intelligence. Dr. Chen was a recipient of the 2016 Outstanding Electrical and Computer Engineers Award from his alma mater, Purdue University (in 1988), after he graduated from the University of Michigan at Ann Arbor, Ann Arbor, MI, USA in 1985. He was the IEEE Systems, Man, and Cybernetics Society President from 2012 to 2013, the Editor-in-Chief of the IEEE Transactions on Systems, Man, and Cybernetics: Systems (2014-2019), and currently, he is the Editor-in-Chief of the IEEE Transactions on Cybernetics, and an Associate Editor of the IEEE Transactions on AI, and IEEE Transactions on Fuzzy Systems. He was the Chair of TC 9.1 Economic and Business Systems of International Federation of Automatic Control from 2015 to 2017 and currently is a Vice President of Chinese Association of Automation (CAA).
内容简介:In this talk, a kind of flat neural networks called Broad Learning System (BLS) is employed to derive two novel learning methods for text classification, including Recurrent Broad Learning System (R- BLS) and LSTM-like architecture: Gated Broad Learning System (G-BLS). The proposed two methods possess three advantages: i) higher accuracy due to the simultaneous learning of multiple information, even compared to deep LSTM that extracts deeper but single information only; ii) significantly faster training time due to the non-iterative learning in BLS, compared to LSTM; iii) easy integration with other discriminant information for further improvement. The proposed methods have been evaluated over 13 real-world datasets from various types of text classification. From the experimental results, the proposed methods achieve higher accuracies than LSTM while taking significantly less training time on most evaluated datasets, especially when the LSTM is in deep architecture.